
Monday, April 27, 2009
Efforts to Defeat Roll-Off

Tuesday, April 14, 2009
Roll-Off, Straight Ticket Voting, and African Americans
Overall
1. There is a significant positive correlation between AATOcomp and STVrate. This causes issues in examining the effect of AATOcomp on roll-off due to the necessary relationship between STVrate and roll-off.
On Partisan Races
2. Without controlling for STVrate, higher levels of AATOcomp are associated with higher amounts of roll-off in top ticket races but this effect reverses as we go down the ticket and there is more overall roll-off. The effect is significant but weak at the top of the ticket, but becomes much stronger down the ballot (presumably as a result of a higher proportion of the remaining votes coming from STV).
3. By subtracting STV vote totals from the overall totals, we can examine roll-off among non-STV ballots.* Using this method, we find that the same positive association between AATOcomp and roll-off exists still in the upper ticket. Continuing to control for STVrate, as we progress down the ticket, we see that there becomes no association at all between AATOcomp and roll-off – with one exception. After the judicial contests, there is the Soil and Water Conservation District Supervisor. In this race there is a very strong positive exponential effect between AATOcomp and roll-off (controlled for STV).
On Judicial Races
4. Surprisingly, there is only a weak positive correlation between judicial roll-off and STVrate. All correlations are positive with bivariate regression slope frequentist p-values < .15 but all are > .01 Controlling for the effects of multiple tests, it is difficult to assert this as a strong effect.
5. In the judicial races, there is no correlation between AATOcomp and roll-off. This makes sense when considered in conjunction with the facts that (1) STVrate has a minimal effect on roll-off in the nonpartisan races (see Observation 4) and that (2) this is a continuation of the trend controlled for STVrate as identified in Observation 3.
*It is important to remember here that we can only study the behavior in the aggregate. We can use this method to understand the voting behavior of the county, but not individuals.
Tuesday, February 3, 2009
Cell Phone Only Population
The most interesting thing to me is the staggering difference in sampling errors for each of the groups. Look at how much wider the 90% intervals are for the CPOs than the landliners. I'm not sure what exactly we can take that to be indicative of in the populations, but it certainly is fascinating.
While I want to agree with the results on a gut level, I have some concerns about the structure of his model. When I checked out the STATA output Schaffner provides, one thing that stuck out to me was the p-value of .845 on the age variable predicting the McCain/lean outcome relative to the base of Obama. I'm not sure if there might be some multicolinearity issues here. I'm going to download the data myself and play around some. I'm off to lunch now, but soon I will revisit this. I'll report back later.
Sunday, February 1, 2009
Senate Debate on Defense
Saturday, January 31, 2009
America's Most Accurate Pollster
Investor's Business Daily put out an editorial declaring their IBD/TIPP poll the best in the nation - again. They called the popular vote margin to the decimal in 2008 and were the closest (of by 0.3%) in 2004. I extend all due congratulations - particularly in an astute allocation of the undecideds, but I just love this link...
Well they certainly were unique in calling the 18-24 year old vote for McCain 74%-22% in late October. Campus was CRAZY that week...
Saturday, January 24, 2009
Cell Phones and Surveys
1. The six versus nine point margin difference between the landline only sample and the complete one with cells is not statistically significant.
2. Weighting the landline sample to the age composition of the exit poll takes care of the discrepancy.
Both of these points are flawed. On the first point, I doubt that the difference is insignificant. The daily margin of error might be three points but these numbers are from aggregated tracking. The margin of error has to be pretty small. But even so the argument is flawed. If I didn't know the results but you asked me which candidate's supporters would be under-represented in a landline only sample, I would be a fool not to pick Obama. The margin of error is a function of the random and unbiased variation we can expect from the nature of proper sampling. Since we can predict the direction of effect of not sampling cell phones, it is specious to compare the size of the effect to the unbiased error we can expect to see. The margin works both ways. One day the sample might advantage the Democrat, and the next it could be the Republican. In a given election cycle, the cell vote does not change direction randomly as a bloc (per the currently accepted theories of the cell vote). Adding in the cell sample changed the margin of victory by three percentage points. That's a decent amount.
In sum, error is random, and bias is not. It is senseless to compare one to the other to downplay its importance.
The second point is a dangerous one. It leads to practices that cause inaccuracy. It trends toward the idea that undersampling and weighting to counter is always an acceptable way of correcting for a cell bias. First, putting a particular weight on cell user turnout is a tricky game. Age corrected the discrepancy for McCain-Obama, but what about other candidate pairs, issues and other poll questions? Second, it justifies undersampling that leads to absurd results. Check that link out. McCain beating Obama 74-22 in the 18-24 vote? There's no way.
Thursday, December 18, 2008
Challenged Ballots in Minnesota
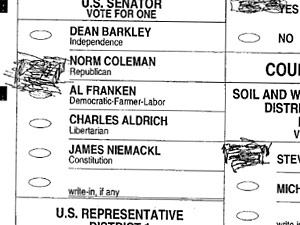